Ma, Ji 马季
The University of Texas at Austin, LBJ School of Public Affairs
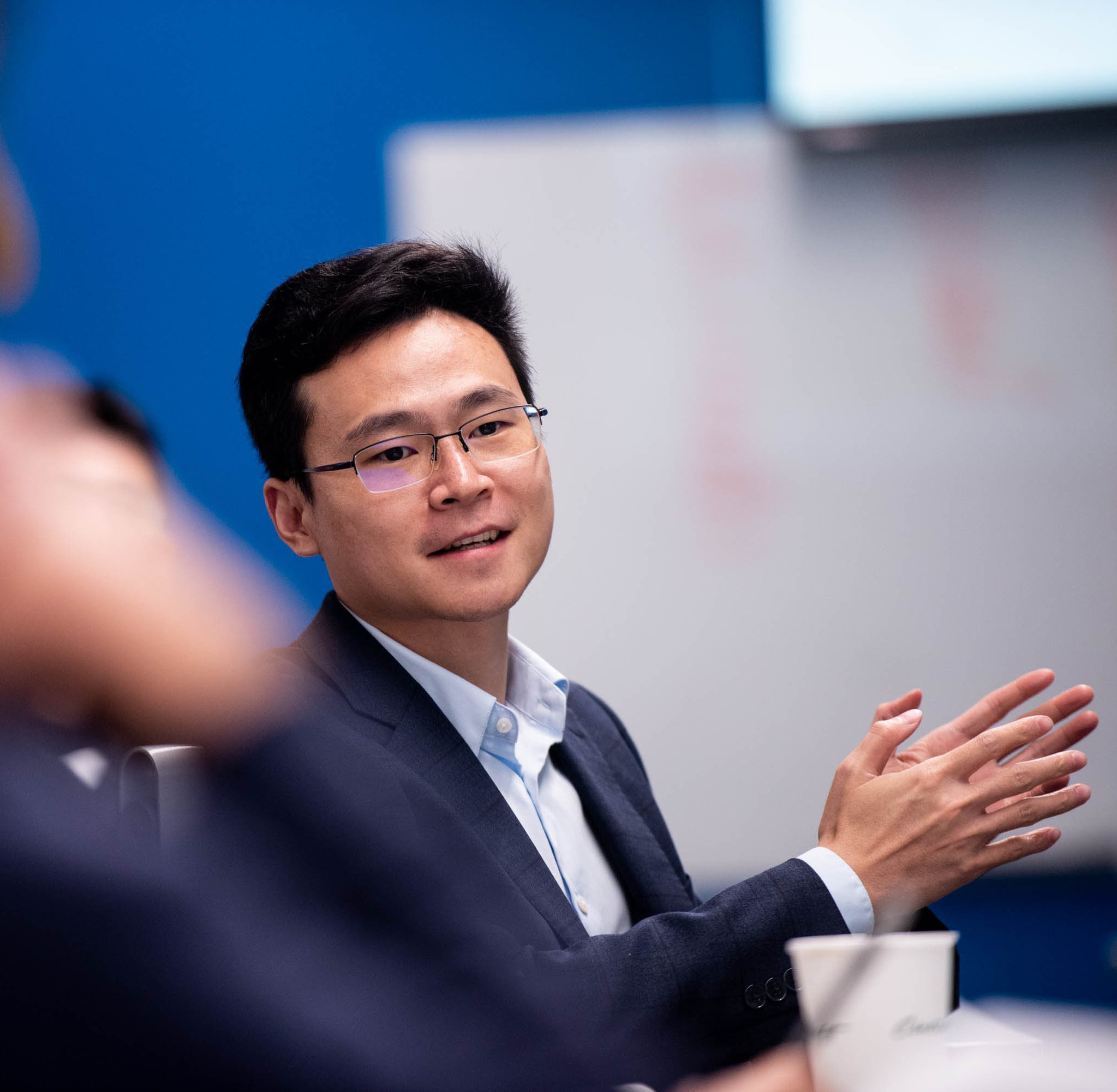
- Office hour: Tue 2pm–4pm (2025 spring).
- Office: SRH3.324, +1-512-232-4240
- Email: [email protected] (preferred) | [email protected]
My name is Ji MA (马季; first name pronounces “G”), Assistant Professor at the Lyndon B. Johnson School of Public Affairs at the University of Texas at Austin. I’m also an affiliated faculty member of the Center for East Asian Studies and the School of Information at UT Austin.
I focus on the nexus of technology and society through three interrelated research streams: (1) using data and algorithms to bring advanced technologies and methods into the public and nonprofit sectors, equipping scholars and practitioners with cutting‑edge tools to address social issues; (2) promoting interdisciplinary and equitable knowledge production for policymaking; and (3) analyzing the resilience and evolution of civil society in restrictive contexts via novel computational methods, thereby generating new theoretical perspectives and research avenues. My publications appear in leading journals across a broad range of disciplines, including public administration and policy, nonprofit studies, sociology, political science, and data science.
In my spare time, I enjoy building open-source projects, tasting tea and hiking with family and friends, and practicing Olympic Recurve.